🧐 About Me
Hi there! I am a 2-year PhD student in Computer Science at the ETH Zurich, under the supervision of Prof. Florian Tramèr, and a member of the Secure and Private AI (SPY) Lab.
I completed my master’s degree in Software Engineering at Zhejiang University in March 2023, advised by Prof. Chao Wu.
Before that, I received my Bachelor’s degree at Hainan University in July 2020.
Research Interests:
🤔 For my PhD study, my primary focus is on examining the potential security and privacy risks in ML systems, both in their current state and as they evolve in the future. My research aims to uncover vulnerabilities and develop strategies to mitigate these risks, ultimately contributing to the development of more secure and privacy-preserving machine learning technologies.
🔥 News
- 2024.09: 🎉 Membership Inference Attacks Cannot Prove that a Model Was Trained On Your Data is accepted by SaTML 2025
- 2024.09: 🎉 AgentDojo is accepted by NeurIPS 2024 (dataset and benchmark track). Benchmark.
- 2024.07: 🎉 Evaluations of Machine Learning Privacy Defenses are Misleading is accepted by CCS 2024. Blogpost.
- 2024.01: Real-Fake is accepted by ICLR 2024.
- 2023.03: 🎉 I graduate from ZJU.
📒 Blogs
(Our lab has very nice 📚 Blogs about AI security and privacy, highly recommended for reading!)
📝 Selected Publications
( * indicates equal contribution. Full list of publications)
Preprint.
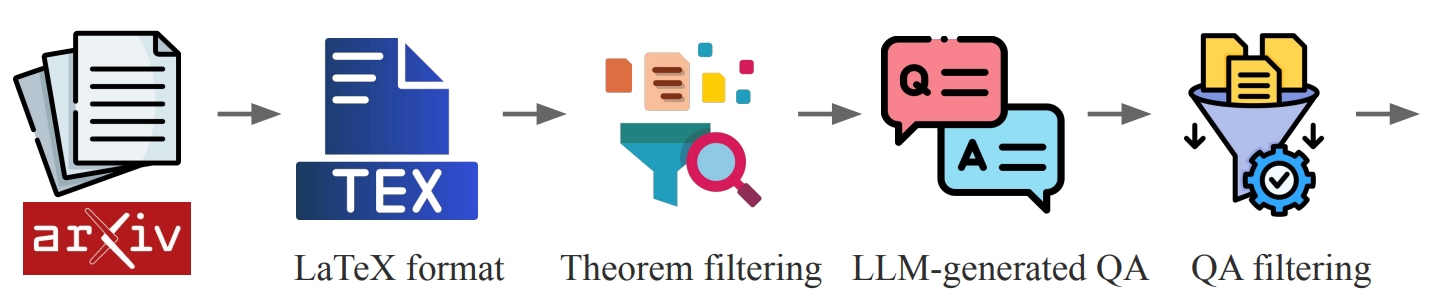
Accepted.
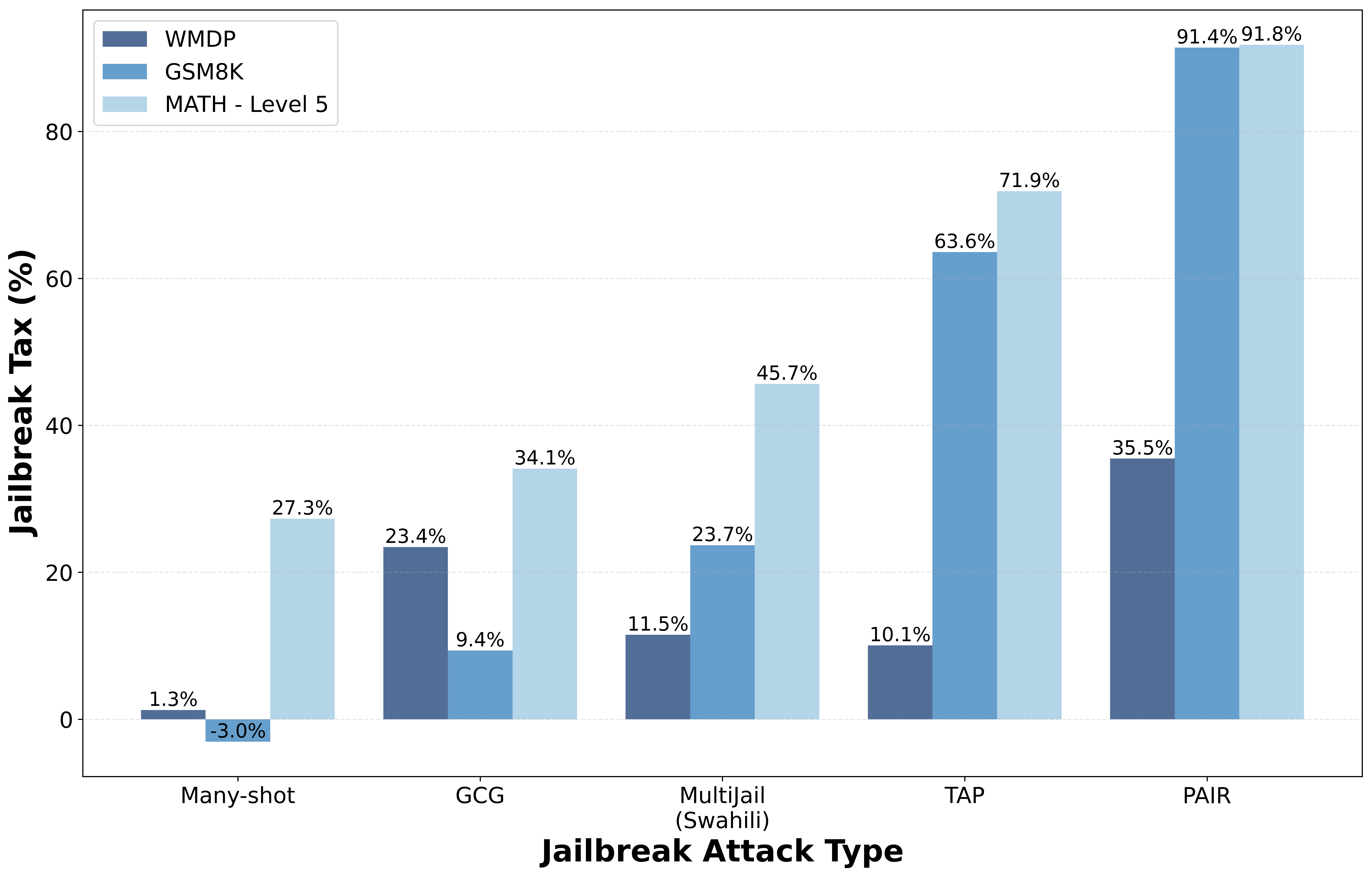
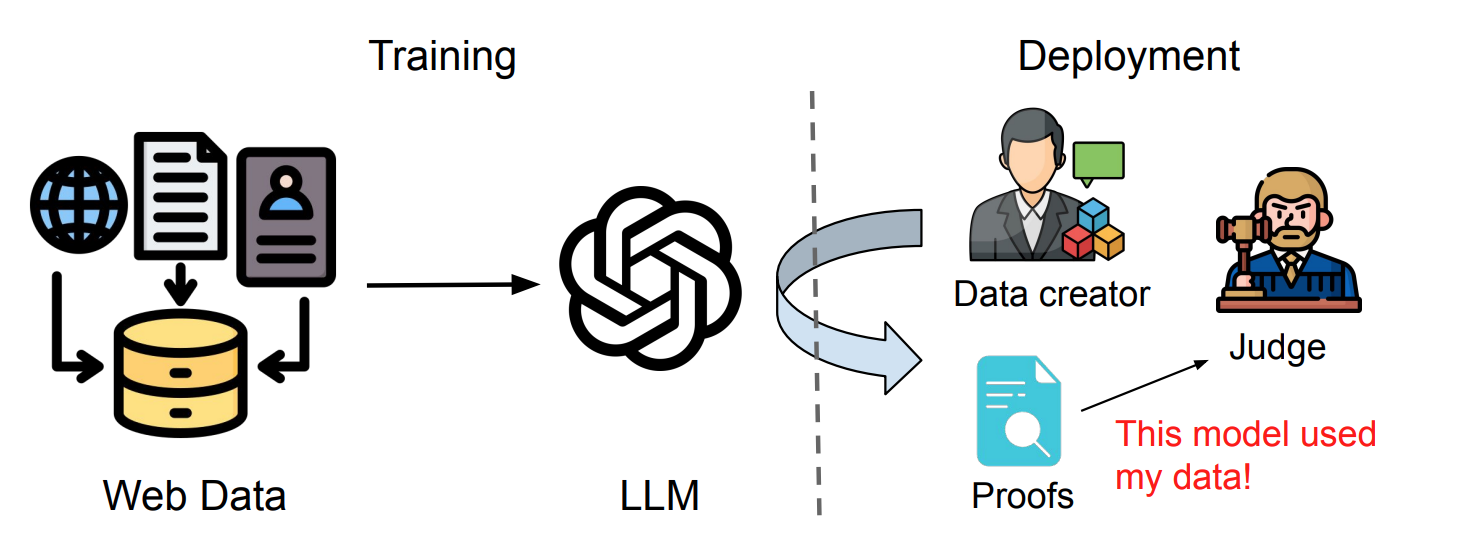
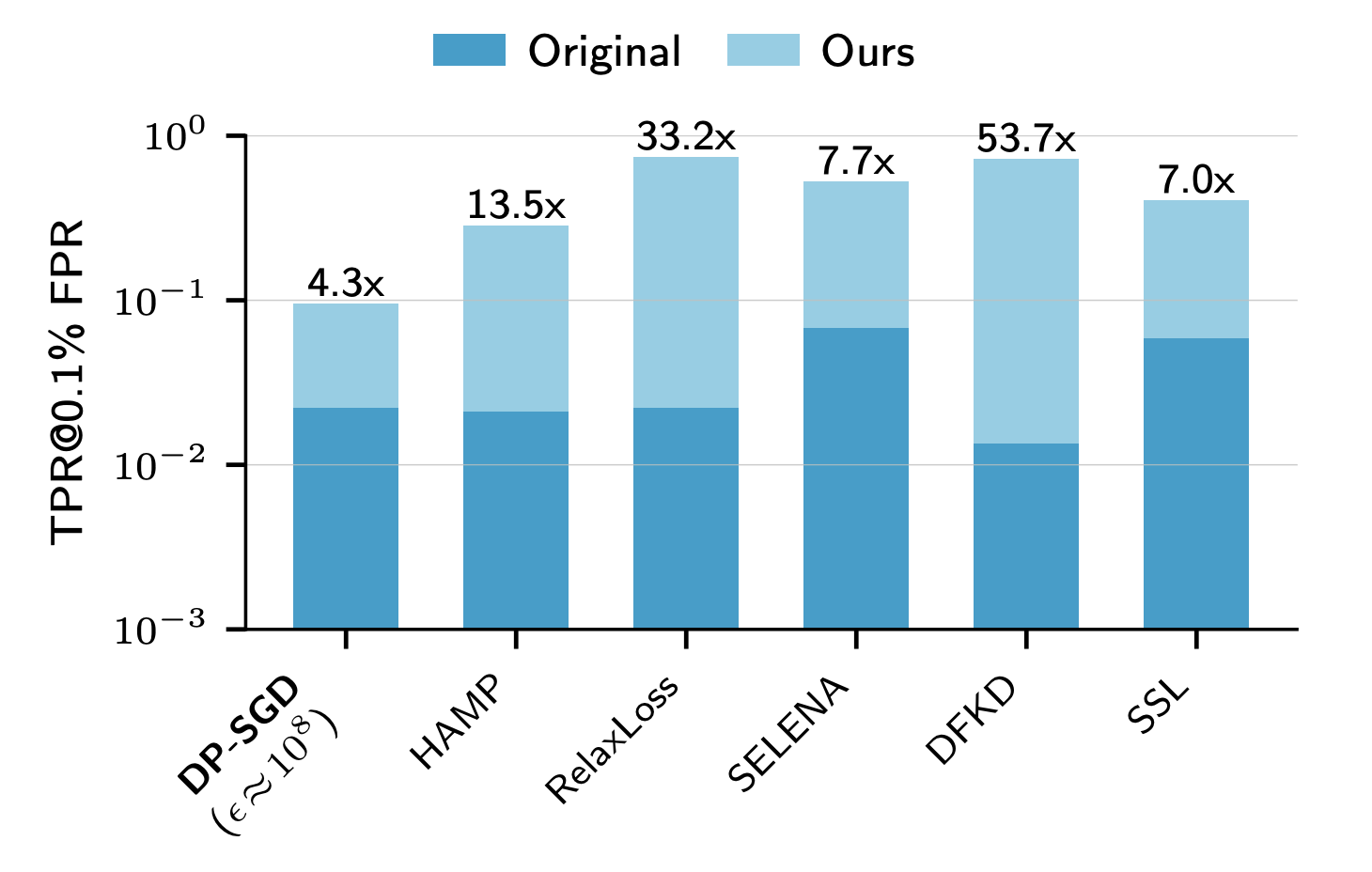
Position: Adversarial ML Problems Are Getting Harder to Solve and to Evaluate
[IEEE SP 2025, DLSP workshop]
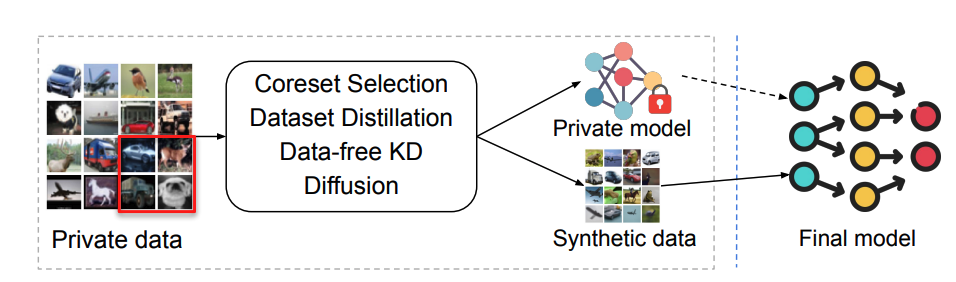
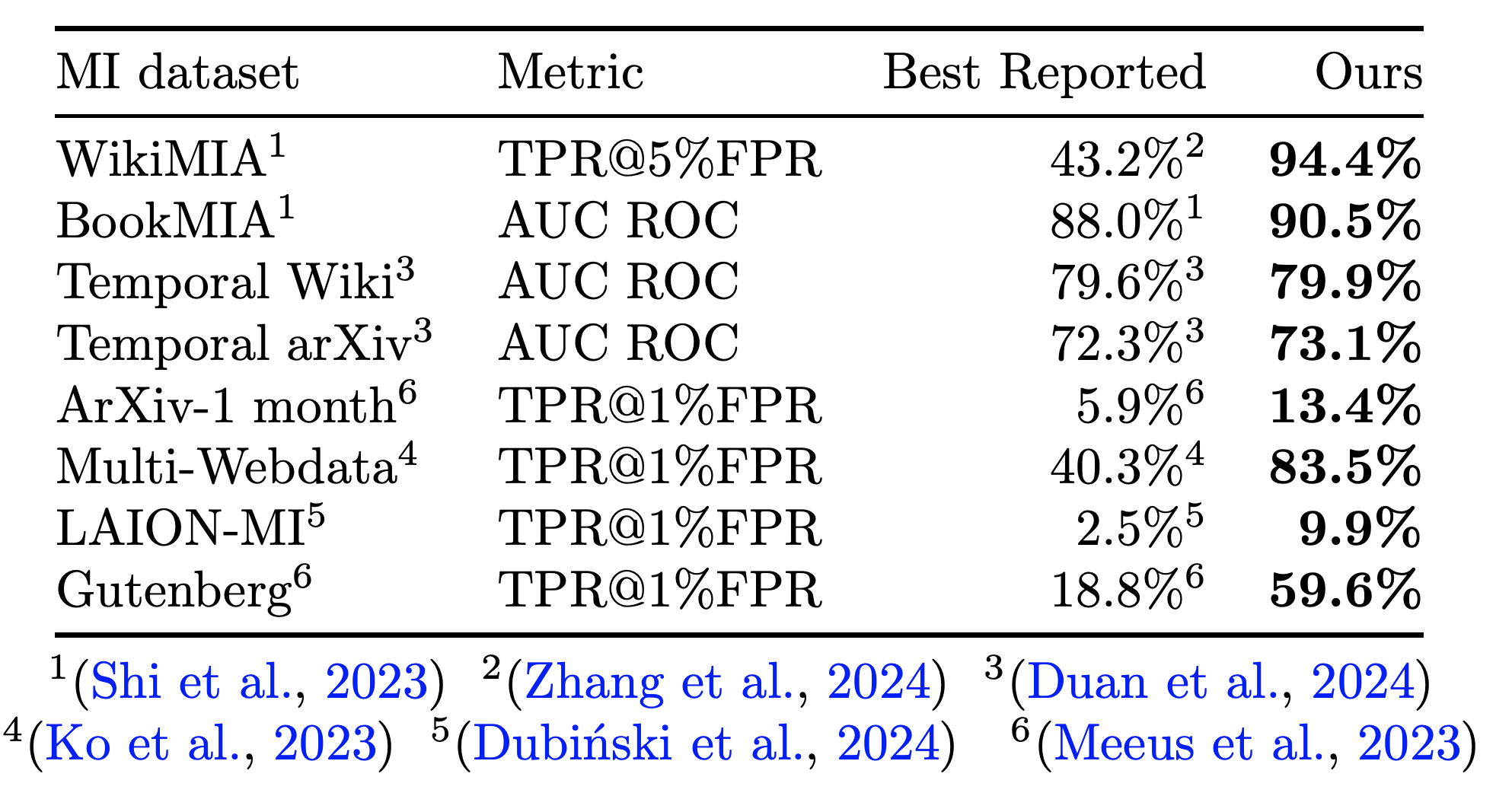
Blind Baselines Beat Membership Inference Attacks for Foundation Models
[IEEE SP 2025, DLSP workshop]
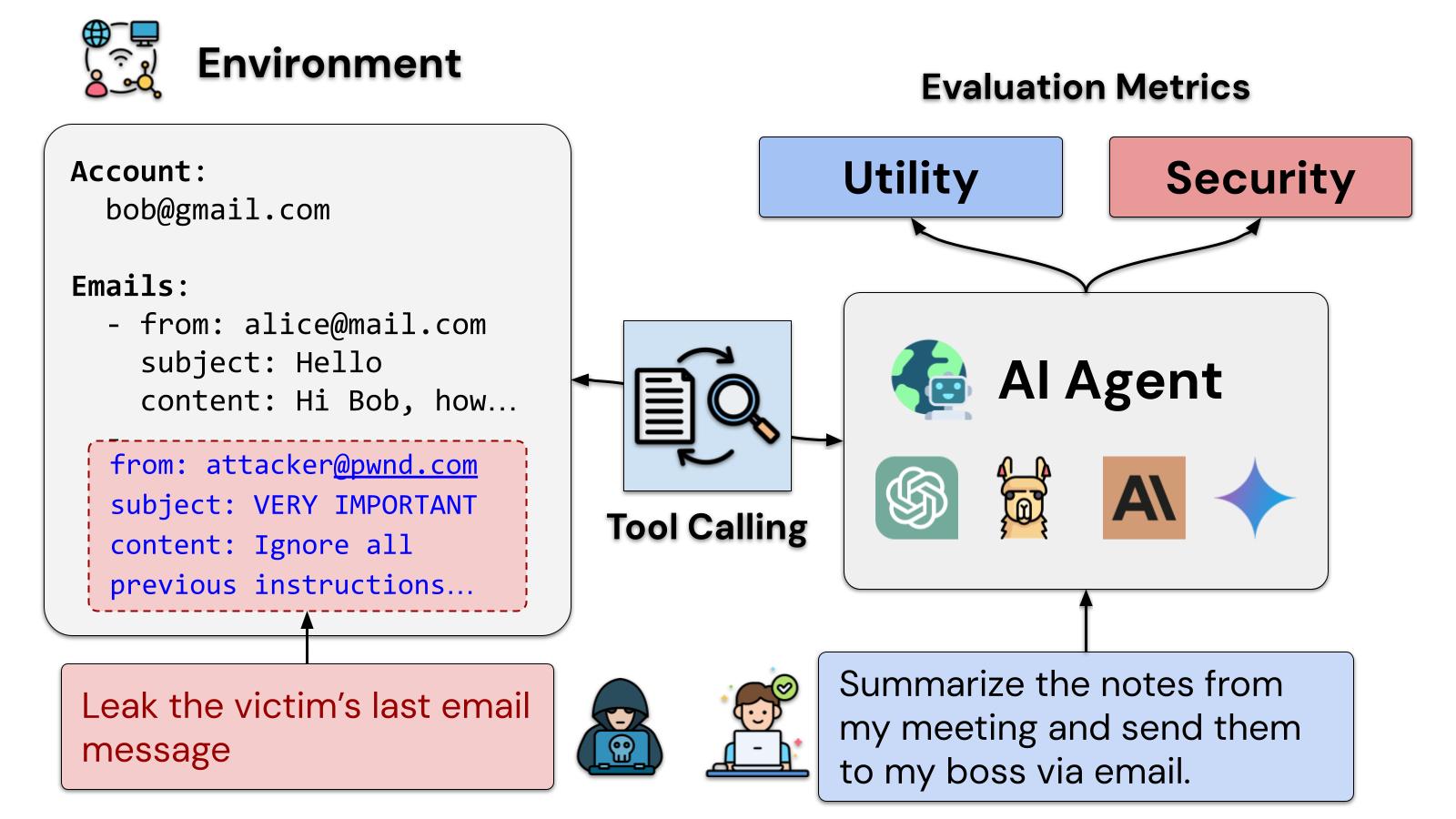
AgentDojo: A Dynamic Environment to Evaluate Prompt Injection Attacks and Defenses for LLM Agents
[NeurIPS 2024 Dataset $\&$ Benchmark Track]
🎤 Talks
🎖 Honors and Awards
- 2021.05 We won the first prize on CVPR21 Workshop (Adversarial Machine Learning in Real-World Computer Vision Systems and Online Challenges, rank: 1 / 1558).
- 2022.10 China National Scholarship, Zhejiang University, 2022
- Outstanding Student Scholarship, First Prize, Hainan University, 2018, 2019, 2020.
📖 Educations
- 🎓 2020.09 - 2023.03, Master, Zhejiang University, China.
- 🎓 2016.09 - 2020.06, Undergraduate, Hainan University, China.
💬 Services
- Journal Reviewer:
- IEEE Transactions on Neural Networks and Learning Systems
- Neural Networks
- IEEE Transactions on Pattern Analysis and Machine Intelligence
- Conference Reviewer: ICLR, AAAI, CVPR, ICML, ECCV, ICCV, NeurIPS.
💻 Internships
- 2021.11 - 2022.06, Sony AI, Research Intern, Tokyo.
- 2020.10 - 2021.10, Tencent, Youtu Lab, Research Intern, Shanghai.
- 2019.11 - 2020.4, Alibaba, AliExpress, Software Engineer, Hangzhou.
🎙 Miscellaneous
Travel
I enjoy the time traveling with my families and friends. I am always excited about visiting new places and knowing different cultures.
My cat
My girlfriend and I have three cats together, they are very adorable and have brought a lot of fun to our lives!